Battery Thermal Management in Formula E
MARCH 6, 2018
Energy strategy in Formula E is a major performance differentiator for race teams and getting this right is a great way to start your battle for the podium. However, at warmer races, not only do teams have to contend with the demands of dealing out the limited battery energy supply, but also with battery overheating. The following extract from the FIA Formula E website explains the situation very clearly:
For the car to be the quickest, the teams and drivers must keep the battery at the optimum temperature through at race, from start to finish. The maximum temperature the battery can function at efficiently is approximately 57 degrees C. It doesn’t take long for these batteries to warm up to that, especially when the ambient temperature is 36 degrees and track temperature 50 degrees as we experienced in Malaysia.
Overheating is a major concern and one of the biggest limiting factor of electric racing at the moment. To protect itself and the driver (and anyone else for that matter) the battery will start to automatically shut down above certain temperatures. In exactly the same way your smart phone protects itself from hot or cold environments by automatically switching off….
…it approximately equates to losing 10 per cent of power for every one degree over the maximum temperature.
Dynamic Lap is already being used by Canopy equipped Formula E teams to generate optimal energy deployment profiles. This powerful tool contains no built-in strategies, which is essential if you want to derive the mathematically optimal energy deployment (for further information see Dynamic Simulation). Put simply, if you feed your simulation with preconceived ideas it will bounce these back at you, but if you want to learn new techniques it’s better to start with a clean sheet of paper.
Having the perfect solution for a single lap is a good start, but the problem with Dynamic Lap is that it has no knowledge of battery thermal issues which might occur 16 laps later. We need the perfect solution for the entire stint which takes all of these issues into account. That’s why we produced Dynamic Multi-Lap!
If you have access to some very powerful computing, you can calculate the deployment strategy for an entire stint. Add in a battery thermal model, and some derate conditions which represent how the battery performance changes with temperature and state of charge and you can simulate the perfect strategy for the entire race:
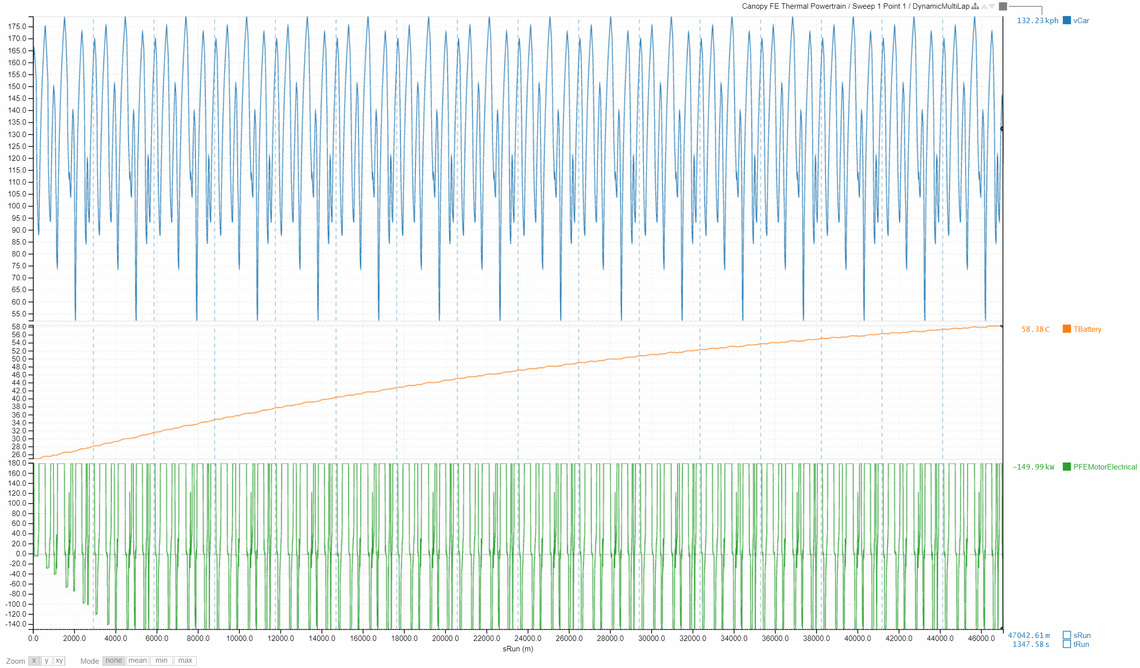
Figure 1: Dynamic Multi-Lap with Thermal Powertrain for race stint.
Unfortunately I couldn’t share a potentially race winning strategy, so this particular Dynamic Multi-Lap has the battery thermal derate conditions removed. When the battery performance is included there are some interesting and elaborate strategies. Our experience has shown that it is very difficult to deduce these through intuition so you’ll have to contact us if you want more details!
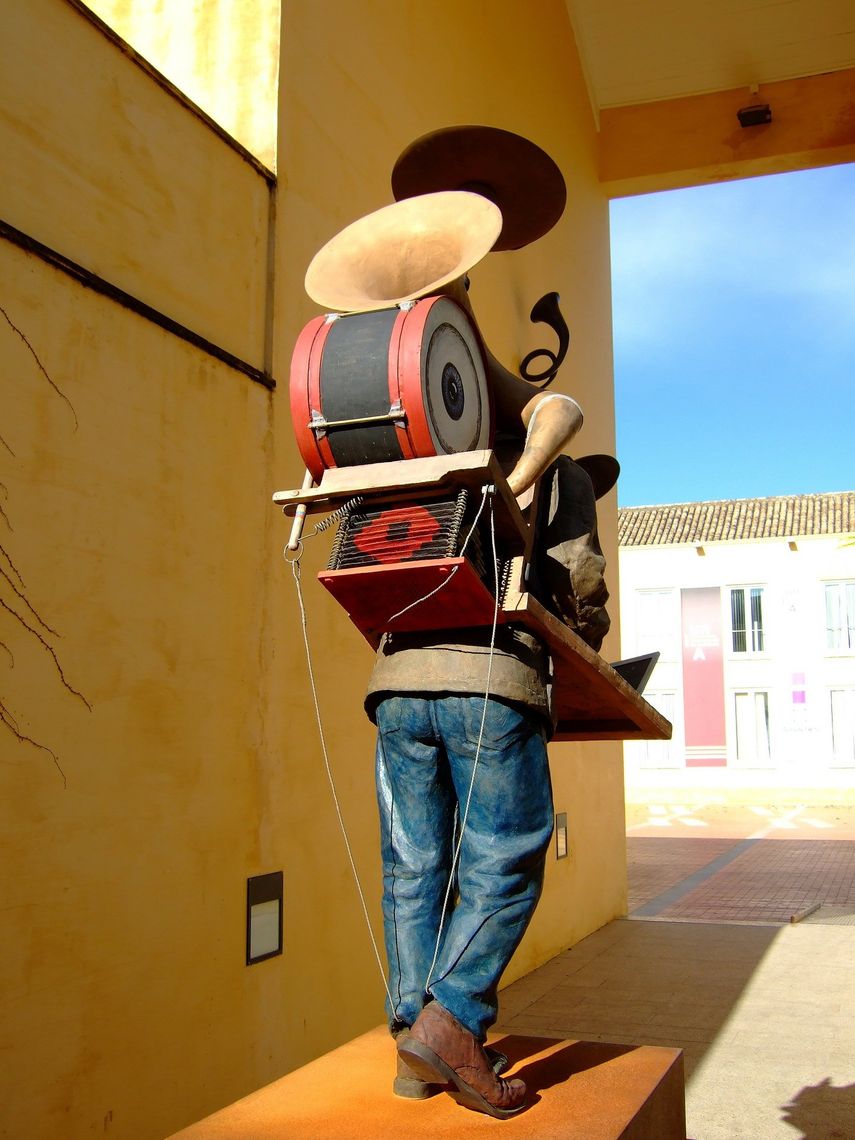
As elegant as this continually adapting strategy is, it gives the teams a massive headache when it comes to ensuring their drivers replicate the important features. It’s already enough of a juggling act for drivers to lift off the throttle at a specific point, then control the regen paddle, and brake at different points for every corner of the lap, before the race engineer comes along to tell them they need to vary this technique for every single lap plus add in a whole bunch of extra tricks for good measure!
Before we ask the driver to perform as a one-man band, let’s find out how much we stand to gain.
To do this we need to compare a continually adapting strategy to a fixed one that is the same each lap. To find the perfect continually adapting strategy we take the following steps:
Adaptive Strategy (Dynamic Multi-Lap)
Correlate the thermal battery model to track data using an exploration of parameters; we run through thousands of combinations of efficiency, thermal, and cooling options in minutes to find the best match. We use a tool called Thermal Replay to emulate battery temperatures from track inputs to facilitate this process.
Input the derate conditions which describe how the battery performs at different states of charge and temperatures.
We’re then ready to run Dynamic Multi-Lap, which runs through the entire stint optimising the overall laptime by changing minute details on every single lap; on lap 1 it already knows that we’re going to run into temperature problems later in the stint, so it can optimise this lap with perfect hindsight!
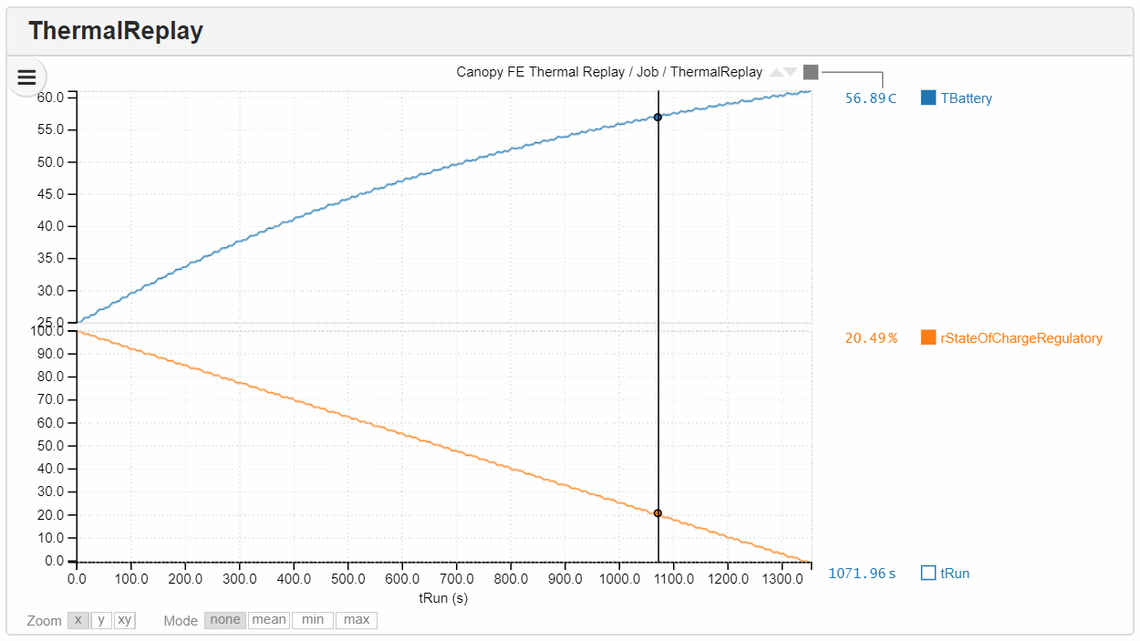
Figure 2: Thermal Replay — emulate your powertrain temperatures using this quick and easy tool.
Fixed Strategy Every Lap (Dynamic Lap)
It is more difficult to find a good strategy if we limit ourselves to a fixed deployment profile on every lap. We found a satisfying procedure to produce these results, however, the estimate comes with a level of uncertainty, hence we have given the laptime estimate an upper and lower bound.
Results
We repeat both procedures for a range of weather conditions (ambient air temperature) so we can prepare for unexpected weather at the race. Now we’re in a position to compare the two strategies:
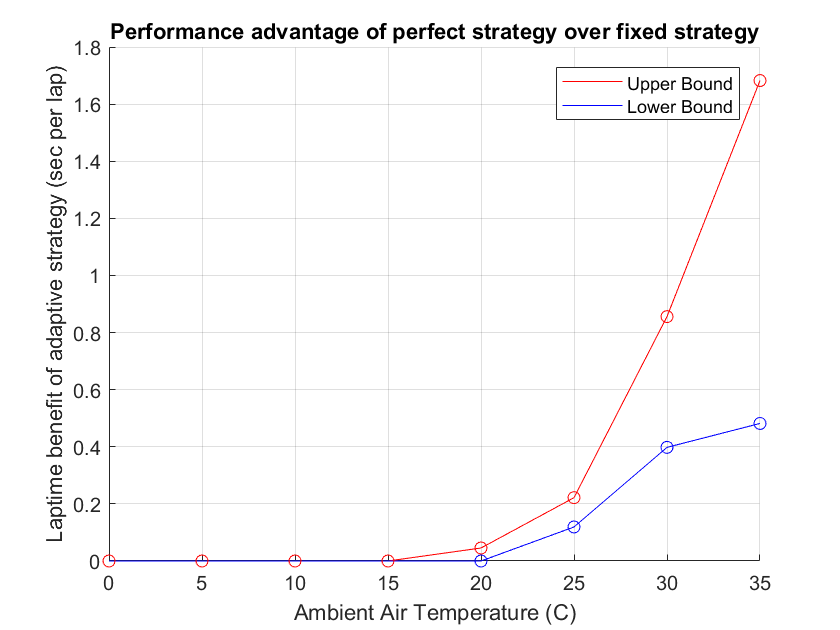
Figure 3: Advantage of adaptive strategy over fixed strategy every lap.
This tells us two really useful things:
If we’re not battery temperature limited, in this case at or below 15C air temperature, then the fixed strategy and adaptive strategy yield similar laptimes.
Once we get to a race situation where we are temperature limited, in this case above 15C, the adaptive strategy (Dynamic Multi-Lap) shows a clear advantage.
In this example, at 30C air temperature we are around 0.63sec a lap quicker with the Dynamic Multi-Lap solution (average of the upper and lower bound). In a warm Marrakesh ePrix that would equate to 20.8sec which could be the difference between winning and being completely out of the points.
We should add that in this example we used a very simple set of battery derate conditions. If we include all the battery characteristics then the optimisation problem becomes more difficult, and Dynamic Multi-Lap sees further benefits. Essentially, the harder the problem, the more you need full stint optimisation to figure it out!
Brake Balance Optimisation
Just when you thought we couldn’t throw any more complexity into the mix for multi-lap simulation, we recently added some extra functionality: brake balance optimisation. This feature allow Dynamic Multi-Lap to automatically adjust the hydraulic brake balance once per lap.
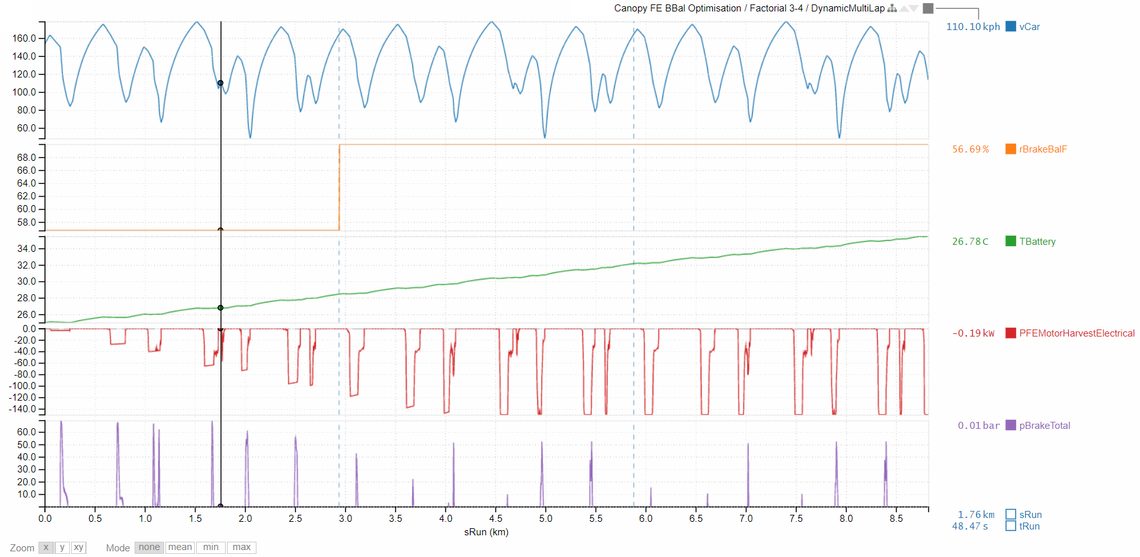
Figure 4: Brake balance (orange) optimised for every lap.
Where the regen power is limited, for example in the early laps of the race when the battery is too full to accept additional charge, the driver moves the brake balance rearwards to make up for the loss of electrical recovery from the rear axle (as shown in Figure 4). While this behavior might seems obvious, where the race is temperature limited we start to see some funky brake balance strategies take place. This pushes the advantage of full stint optimisation one step further.
Conclusion
Energy simulation in Formula E is already enough of a challenge, but when the temperature soars, teams find themselves limited by battery performance. Depending on the temperature, the race can fall into one of three scenarios:
Energy limited: we don’t encounter any thermal issues. Dynamic Lap can be used to find the optimal deployment.
Energy & temperature limited: thermal issues force us to change our strategy, but it is still possible to deploy all of the battery energy. Dynamic Multi-Lap is required to optimise both the energy and thermal performance together.
Temperature limited: this leads to the disappointing situation where we are forced to pit early due to thermal issues before we have emptied the battery. Dynamic Multi-Lap will show where it is advantageous to avoid this scenario, and move the race back to scenario 2 where we can still suck all the juice out of the battery.
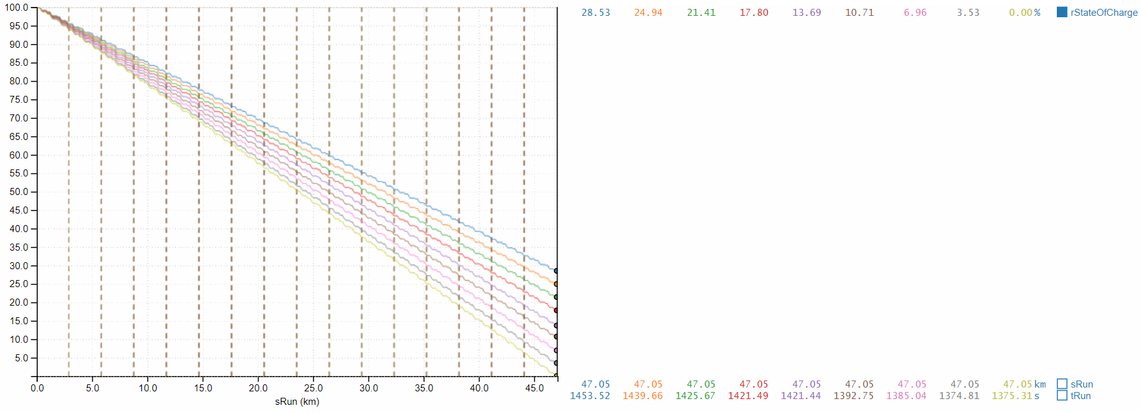
Figure 5: Battery state of charge through the stint. Will your run come to an end before the battery is empty?
Managing the thermal performance efficiently can move teams from outside of the points to the top step on the podium. Fortunately we’ve made this complex optimisation problem relatively simple through the use of Dynamic Multi-Lap. If you would like to speak to us about thermal optimisation (powertrain, tyres or otherwise) then please get in touch at hello@canopysimulations.com.